
We discuss various data sources and representations that affect the resulting generated content.
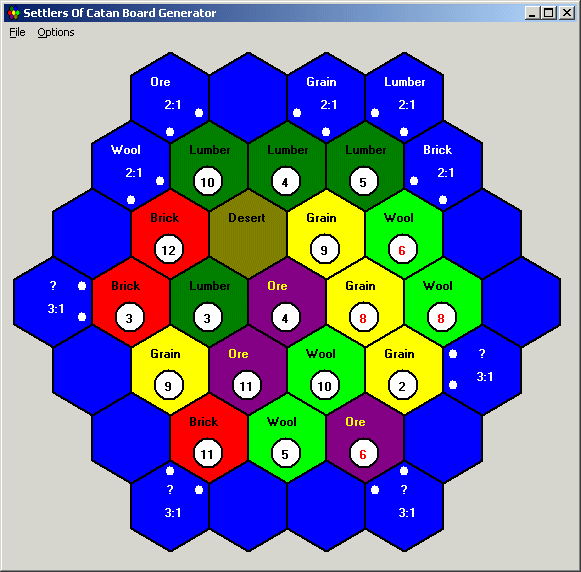
In addition to using PCG for autonomous generation, co-creativity, mixed-initiative design, and compression, PCGML is suited for repair, critique, and content analysis because of its focus on modeling existing content. We focus on what is most often considered functional game content such as platformer levels, game maps, interactive fiction stories, and cards in collectible card games, as opposed to cosmetic content such as sprites and sound effects. As the importance of PCG for game development increases, researchers explore new avenues for generating high-quality content with or without human involvement this paper addresses the relatively new paradigm of using machine learning (in contrast with search-based, solver-based, and constructive methods). This survey explores Procedural Content Generation via Machine Learning (PCGML), defined as the generation of game content using machine learning models trained on existing content.
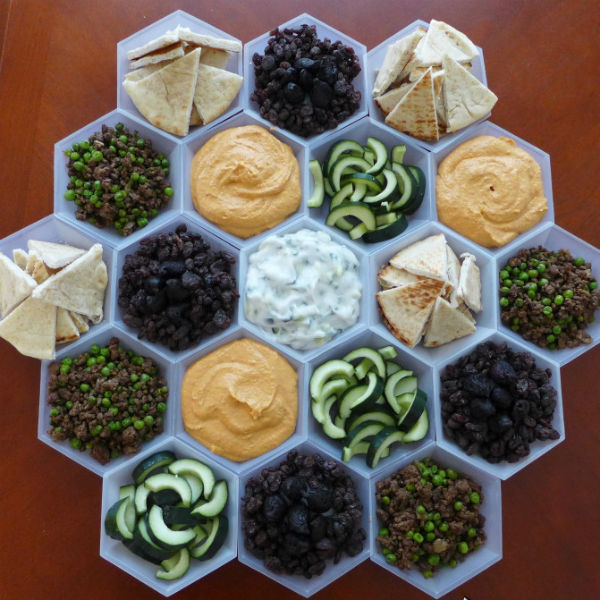
We see the analysis performed here as a possible template for AI-based playtesting of contemporary board games. Finally, the automated analysis also reveals two classes of failures states, where the agents find states which are not covered by the game rules this is akin to finding bugs in the rules. Further analysis of the automatically played games reveal which cities on the map are most desirable, and that the relative attractiveness of cities is remarkably consistent across numbers of players. The performance profiles of the different agents can be used to characterize maps and identify the most similar maps in the space of playstyles. Different playing styles are shown to be effective depending on the map and rule variation, and also depending on how many players play the game. In this paper, four different game-playing agents that embody different playing styles are defined and used to analyze Ticket to Ride. Ticket to Ride is a popular contemporary board game for two to four players, featuring a number of expansions with additional maps and tweaks to the core game mechanics. In particular, we identify the different creative facets of games, we propose how orchestration can be facilitated in a top-down or bottom-up fashion, we review indicative preliminary examples of orchestration, and we conclude by discussing the open questions and challenges ahead. What happens, however, when the creative process involves more than one creative domain such as in a digital game? How should the different domains influence each other so that the final outcome achieves a harmonized and fruitful communication across domains? How can a computational process orchestrate the various computational creators of the corresponding domains so that the final game has the desired functional and aesthetic characteristics? To address these questions, this article identifies game facet orchestration as the central challenge for AI-based game generation, discusses its dimensions and reviews research in automated game generation that has aimed to tackle it. When the process is based on a single creative domain such as visual art or audio production, designers primarily take inspiration from work within their domain and refine it based on their own intuitions or feedback from an audience of experts from within the same domain. The design process is often characterized by and realized through the iterative steps of evaluation and refinement. Mathematics of computing → Combinatorial optimisation.Al-gorithmic game theory and mechanism design → Representations of games and their complexity.Mathematical optimization → Bio-inspired optimization.Design and analysis of algorithms → Evolutionary algorithms.Moreover, the results showed to be promising when compared with existing game maps and setup. The resultant configurations satisfy complex game-dependent requirements while optimising a model for game balancing. This paper investigates the application of the ant colony optimisation metaheuristic to generate content and improving game balance for two well-known tabletop games, namely, Terra Mystica and Settlers of Catan. The use of such methods for tabletop games, however, has been scarce in the academic literature, for the best of the authors' knowledge. The literature contains examples of methods that employ artificial intelligence to generate maps in computer games that offer balanced and fairness of starting conditions for the players.

Approaches for evaluating and achieving game balancing include extensive playtesting-which typically requires several iterations of games with subtle adjustments in the components and adopted strategies that resemble brute force-and algorithmic solutions that use qualitative and measurable design goals when developing game components. Game balancing is one of the most challenging features to be implemented in a typical game design process.
